A/B Testing 101: What It Is And How to Do It Right
2024-05-01
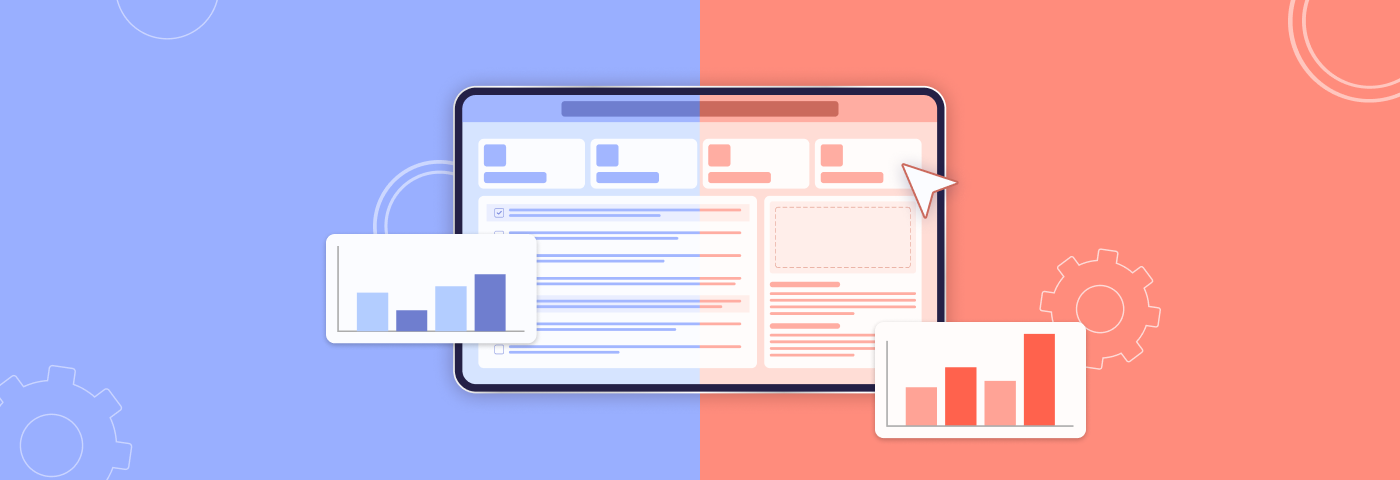
Discovering what resonates with your audience is key to advertising and marketing success. That’s where A/B testing comes into play: it allows you to make informed decisions and optimize your strategies for better results.
In this guide, we’ll dive into the details of what is A/B testing, how you can benefit from it, and how to do it right.
What is A/B testing in marketing and advertising
In simple terms, A/B or split testing is the process of comparing two or more versions of something to determine what works better for a specific purpose. In advertising, this usually includes various elements of an ad: headlines, visuals, CTA buttons, and so on. You show version A to one group, version B – to another, and analyze which one gets a better reaction from your audience.
A is the 'control' version, or the original testing variable. B is the 'variation', or a new version of the original testing variable.
The logic of A/B testing is simple: a change in a variable can hypothetically cause a change in metrics. The goal of testing is to figure out whether particular KPIs will improve depending on specific changes in the variable.
Besides A/B tests, there are other types of tests that you can run:
A/B/n tests
A/B/n tests allow you to experiment with multiple variations within one hypothesis: you can have not just A and B variations but C, D, E, etc. – however many you need. This helps marketers to identify the best-performing option more efficiently than A/B testing alone.
Keep in mind that A/B/n tests require higher capabilities for a proper testing process: you’ll need a larger audience to achieve the right sample size, more traffic to split evenly between the pages, and so on.
A/A tests
The main goal of A/A tests is to compare the same variation against itself. The audience is divided into two groups, but both groups are exposed to the same version of the variable being tested.
A/A tests are usually done as a part of A/A/B testing for validation purposes: you compare two identical variants (A and A) against a third variant (B). This helps eliminate all other possible factors that could influence the results except for the changes in a specific variable.
If there are no significant differences between the performance of identical versions for each part of the audience, it means that the A/B testing results were correct and one variation of the variable – A or B – performed better than the other.
Multivariate tests
Multivariate tests also compare the control version against multiple variations just like A/B/n tests, but the process involves testing multiple elements or variations simultaneously.
This helps to determine the combined impact of different factors. For example, you can test several variations of a landing page where each element (e.g. a CTA button) will have different versions. Ultimately, you’ll be comparing different combinations of elements against each other.
Multivariate tests are more complex and resource-intensive than A/B or A/B/n tests – they require higher traffic volumes. However, they help marketers identify the most effective combination of elements and refine their strategies.
Bandit tests
Bandit tests function based on a principle of dynamic traffic allocation: the audience is dynamically allocated to different variations based on their performance during the test. For example, if you use bandit testing for comparing several versions of a landing page, more traffic will be sent to the winning version to avoid losing potential conversion opportunities.
Bandit tests are particularly useful in scenarios where rapid optimization is essential, such as real-time personalization or dynamic content delivery. However, they require careful design and monitoring to balance exploration (trying new variations) with pragmatism (leveraging known high-performing variations) effectively. For the sake of simplicity, we’ll only consider A/B tests in this article as it’s the most widely used testing approach in advertising and marketing. Let’s start by clarifying a few things.
Why do you need A/B testing?
If you’re not regularly conducting A/B tests yet, you’re missing out on multiple benefits that can help you get better results from your marketing and advertising campaigns.
Here’s what you can achieve with A/B testing:
1. Understand your audience
A/B testing provides objective data about what works better for your audience: makes them click, buy, subscribe, read to the end, etc. By analyzing testing results, you can identify core preferences, needs, and behavior patterns of different audience segments – the key to running successful campaigns.
2. Make data-driven decisions
The empirical data you get from running A/B tests allows you to make informed decisions based on measured user responses rather than assumptions. Strategies backed by data bring better results, and A/B testing is the best way to get actionable insights you can put into practice.
3. Increase conversion rates and ROI
Identifying the most effective strategies through A/B testing allows you to allocate your budget to things that deliver the best results. If you scale top-performing variations, you get more conversions, and if you minimize wasteful spending, you get more out of your existing budget.
4. Improve user experience
User experience encompasses all aspects of how users interact with a product, service, or brand: visiting the website, viewing ads, consuming content, etc. It includes steps users take when they move through the conversion funnel.
A/B testing at each of these steps helps to provide user experiences tailored to the journey and expectations of specific groups of customers, which brings them to the desired destination faster.
4. Create better content
A/B testing isn’t strictly limited to marketing and advertising – it can also be used to create engaging content for your audience. Experimenting with different elements of social media posts (headlines, images, length, messaging, etc.) helps to pinpoint what keeps your audience engaged.
If you A/B test regularly, analyze the results, and discover actionable insights based on data, you can upgrade your strategies and gain a competitive edge that will set your business apart from everyone else.
What can you A/B test?
If you’re looking to refine your marketing and advertising campaigns, try A/B testing these elements:
1. Landing pages
Your landing page is the face of your website and business: it’s responsible for users’ first impressions and influences their experience of moving through the conversion funnel. In short, a landing page is either delivering you conversions or not.
You can track these metrics to make sure your landing pages are optimized:
- Bounce rates,
- Average time on page,
- Conversion rates,
- Dwell times,
- Scroll depth,
- Page load time.
To improve these metrics, test these landing page elements:
Offers
Relevant and competitive offers make users stay and explore your website and offers that miss the target lead to website visits that don’t lead to conversions. You can test offers with or without discounts, free trial proposals, additional benefits, and features for subscriptions or purchases of higher values, and so on.
Copies
The main purpose of copywriting is to communicate the unique selling proposition (USP) and the offers of a business – the more it taps into the needs and pain points of the target audience, the better it is at converting visitors into paying customers and subscribers.
You can experiment with the copy’s length and tone of voice, try including humor or emojis in it, and so on. Testing different copies for specific audience segments can help you create personalized landing pages for each group of users based on their characteristics and their stage in the conversion funnel.
Visuals
How a landing page looks is also important: high-quality visuals and matching color palettes can influence the decisions of your potential customers. You can test different visual formats (images vs. videos) and their placements on a page, various color schemes, combinations of graphic and interactive elements, etc.
Layouts
Landing page layouts that follow both the logical structure and the user’s journey through the funnel can maximize engagement and conversions.
Here, you can compare single-column vs. multi-column designs, different placements of elements (headlines, visuals, user reviews, CTAs, etc.), types of navigation, typography and font sizes, and so on.
When A/B testing your landing pages, pay attention to how users access your website, i.e. which devices they use. For more accurate results, test different elements for control and variation groups that use the same device.
2. Emails
Email marketing is a valuable tool for businesses to engage with their audience, nurture relationships, and drive sales. It’s also a ripe ground for testing different approaches to customer acquisition and retention.
To know how effective your email campaigns are, monitor these metrics:
- Open rates;
- Click-through rates;
- Conversion rates;
- Unsubscribe rates;
- Engagement by device.
Here’s what you can test to improve these KPIs:
Subject lines
Most users will decide whether to open an email based on its subject line: if it spikes their interest, they’ll probably check what’s inside the email. You can experiment with different degrees of personalization, lengths, and tones of voice of subject lines, try adding emojis, humor, or numbers to create intrigue, and so on.
Audience segments
Various groups of users can react differently to your emails based on who they are, where they are in your conversion funnel, how they use your products or services, their primary pain points, etc.
Running identical email campaigns for different audience segments can help you measure their reactions and find what strategies work better for specific audience segments.
Content and format
While catchy subject lines may lead people to open your emails, the content and format of the message will determine whether they’ll actually read it. You can test different email lengths and CTAs, experiment with testimonials, reviews, statistics, or case studies, send emails with or without visuals, and so on to see what makes the recipients take the action you want them to.
Timing and frequency
When and how often you send emails is also important for the success rates of your campaigns. For example, your customers can be more likely to open your emails during specific hours of the day depending on what you’re offering – the time periods will be different for B2B and B2C email campaigns.
You can test different hours of the day or days of the week to determine the best times for sending your emails, and test different frequencies to learn how often your customers want to hear from you.
3. Ad campaigns
Running ad campaigns online requires knowing your audience's preferences, best advertising practices in your industry, the specifics of how different platforms work, and so on. A/B testing your ad campaigns can help you figure out the best ad variations that will bring you high ROI.
Here are the ad elements you can A/B test:
Ad visuals
Ad visuals can make or break your campaigns’ success: if your target audience doesn’t find them engaging and relevant, you won’t get the results you’re aiming for. You can test visuals with different colors, elements, and their arrangements.
For example, you can compare the performance of ads with visuals that have people in them vs. visuals that focus on products only, visuals with vs. without text, with vs. without your brand’s logo, and so on.
Ad copies
Text is no less important for ads’ success than visuals. In fact, most people make the decision whether to click on an ad or not based on what it offers, i.e. the copy. You can compare copies of different lengths, with different offers, tones of voice, with or without testimonials, numbers, humor, etc.
If you want to improve your results, check out the best practices and tips for writing effective ad copies we’ve shared before.
CTAs
Call-to-action is a small yet important element of an ad: if it’s not aligned with your audience’s intentions, it’s less likely to inspire people to take action. You can experiment with various placements, sizes, shapes, colors, and texts of your CTAs.
Ad formats and placements
Most advertising platforms provide various formats for creating ads and placements for displaying them. Testing these options allows you to make your ads more tailored to your audience’s preferences and show them in more suitable places.
You can compare the performance of single-image ads vs. carousels, image vs. video ads, and other formats for specific audience segments. This will help you figure out what works better for different groups of customers and different stages of the conversion funnel.
Ad schedules
As with emails, users can be more or less receptive to your ads during specific time periods depending on many factors: who they are, their online behaviors, the products or services you’re promoting, and so on.
Ad running schedules for B2C and B2B businesses can be very different: in one case users will be more likely to click on ads during their leisure time, and in the other, they’ll give more clicks while they’re at work. A/B testing different ad running schedules can help you find time slots with the highest click-through and conversion rates.
Keep in mind that this is far from being a comprehensive list of things you can A/B test. You’ll find more opportunities for testing when you analyze your existing campaigns and figure out what can be changed to make them more effective.
How to A/B test the right way
Now that we know why and what to A/B test, let’s go through the key steps for running tests that give accurate results.
1. Specify your objectives
To run A/B tests properly, you need to understand why you’re doing it in the first place. You can start by answering this question: “What do I want to learn from it?”. Specifying the desired outcome will provide the right KPIs for your test and the basis for formulating your hypothesis. For example, your goal might be to figure out how a particular change in your ad copies or visuals will influence your CTR or conversion rate based on a specific hypothesis.
2. Identify the variable for testing and create A and B variations
To get reliable data from your tests, choose only 1 variable at a time: a headline, a visual, a CTA button, a page layout, etc. Limiting the testing process to just one variable allows you to clearly attribute the changes in your advertising KPIs to a specific factor without confusion.
You can choose something minor as your variable: the color of a button on your website, the tone of voice of a headline, etc. But you can test at greater scales too, comparing two different versions of a landing page, a promotional email, an ad, and so on.
How big or small the testing variable will be depends on your objectives, resources, and prior testing experience. For example, if you’re redesigning your website’s landing page and have two distinct layouts to choose from, it’s better to start by testing them instead of focusing on smaller elements like buttons – they can be tested later if necessary.
To select the right variable, answer these questions:
1) Will it have an impact on key metrics?
Testing just for the sake of it is not only impractical but also consumes a lot of resources and time. That is why it’s crucial to test variables that can actually improve the KPIs integral to your campaigns.
For example, if you're running a traffic campaign and want to increase the number of ad clicks you're getting, you can test two ad copies with distinct discount offers – this is likely to have a measurable impact on ad performance. To make the right choice, you need to clearly formulate and know which variables your audience pays attention to.
2) Can it be tested?
It should be realistically possible to test the selected variable – you should have enough resources and time to properly execute the testing process. It’s best to avoid variables that require extensive resources or prolonged testing periods if there aren’t enough good arguments in favor of testing them.
If you want to make sure that a particular A/B test is justified, consider whether the risks of not testing the variable outweigh the required expenses. For example, you can estimate how many conversions you’re potentially missing out on by not changing ad creatives and decide if testing different variations can ultimately be cheaper than leaving everything as it is.
Once you pick the variable, create the A and B variations by modifying it.
3. Develop a hypothesis
During the A/B test, you’ll have to track the changes of a specific metric and measure them against a defined goal. This is done to check whether the modifications of your variable had a positive, negative, or neutral impact on these changes.
To do all of that, you need to formulate a hypothesis – it will provide a structured framework for your tests. This hypothesis will be compared against the null hypothesis – the presupposition that there’s no relationship between the changes in specific variables and the performance metrics.
The skeleton of your hypothesis can be fairly simple: a shorter ad copy (ver. B) will lead to a higher-than-average CTR than a longer copy (ver. A) with all other ad elements being the same.
A good testing hypothesis should have the following characteristics:
- It is measurable and testable;
- It is formulated clearly and can be easily understood;
- It specifies the KPIs that can be improved (e.g. conversion rate);
- It specifies the audience segment that’s going to see the A and B variations.
Avoid overly broad definitions for your hypothesis: incorporating multiple variables or too many metrics can create confusion, hindering your ability to accurately assess the testing outcomes in the future.
To formulate a clear and testable hypothesis, focus on a specific audience segments: mobile or desktop users, individuals with a particular professional background, certain income level, etc. Being precise allows you to get more accurate results by eliminating all other potential factors of influence.
When your test is over, you’ll be able to analyze the results and make a decisive conclusion about whether your hypothesis was verified or disproven.
4. Choose an appropriate sample size and split it randomly
Selecting the right audience is integral to testing success, and to do so we need to employ a few key concepts from statistics. Realistically, we cannot show our A and B variations to all relevant users, i.e. the population as a whole – it will take too much time and resources to do so. That’s why we use samples instead of populations in statistics and A/B testing.
A sample is a smaller subset of the population that is chosen for observation and analysis to draw conclusions about the entire group. As we established earlier, you need to choose a specific group of users for your test (e.g. users from a particular demographic who view your ads from mobile devices). Then, you’ll select a sample from this population.
A quality sample for A/B testing should be:
1) Large enough
This is crucial because it helps ensure the reliability and validity of the results – they need to be representative of the entire population. A larger sample size reduces the likelihood of random variations influencing the outcomes and provides more accurate insights into the actual impact of changes being tested.
Source: Statsig’s sample size calculator.
If you’re not sure how big your sample should be, use calculators like Optimizely, Statsig, CXL, and others to find out.
2) Randomly selected
Random selection means that each member of the population has an equal chance of being included. This helps minimize bias and ensure that the sample is representative.
3) Randomly split into two groups
Your A and B variations should be shown to two separate groups of users from your sample. For the sake of being representative, it should be randomly split into control and variations groups.
This makes the groups comparable at the start of the experiment and helps avoid skewed results.
5. Run your tests simultaneously and for a specific duration
The performance of marketing and advertising campaigns can vary during different hours of the day, days of the week, seasons, etc. That is why running both A and B variations at the same time is crucial to minimize the influence of time on testing outcomes.
Another time-related rule of A/B testing – running your tests for a sufficient duration of time to gather enough data and minimize the influence of factors not related to the variable on the results. For example, the results during the weekends can differ from what you might get during the week, users can react more positively to novelty at first but not necessarily after some time, and so on.
You might also inaccurately spot “the trends” in users’ reactions to a particular variation if you don’t run your tests for long enough. A better performance of one variation during the first few weeks does not mean that it will stay the same when you finish the test, so it’s best not to rush and make conclusions too early.
In short, give your A/B tests more time for completion, and you’ll get more accurate data as a result. You can follow experts’ advice on A/B testing duration and run tests for at least 4 weeks even if you reach your optimal sample size.
6. Track, analyze, and document the results
You have to make sure that you’re tracking all important events and actions to get accurate and comprehensive data from your tests. Hence why it’s important to choose reliable tracking tools (like Google Analytics or Facebook Pixel) based on what and where you’re testing.
Once the test is finished, you need to compare the performance of A and B variations based on your KPIs to check which variation was closer to the desired outcome. Don’t forget to check for statistical significance: a value of at least 95% will help you make sure that the observed differences between variations are ‘significant’, i.e. valid. You can use online calculators from AB Test Guide, Neil Patel, etc. to calculate the significance of your results.
When checking performance metrics, consider the secondary ones as well: in some cases, checking KPIs that you didn’t include in your test originally might provide additional insights about the influence of specific variables on performance.
The next step after evaluating the results and arriving at the right conclusions is to document the outcomes (including key metrics, performance improvements, and any unexpected findings) and share them with relevant teams. No matter how big your organization is, anyone who might benefit from knowing the A/B testing results should be made aware of them.
7. Iterate and repeat
Finally, you’ve learned that the variation (B) performed better than the control version (A) and it was because of specific changes in certain variables. Congrats! You’ve finished your A/B test and confirmed your hypothesis.
But that’s not all: now you have to use the insights you’ve got from A/B tests. With this data, you have the opportunity to apply the changes that led to better performance and check if similar changes can be made for other ads, landing pages, emails, etc. When you get fresh ideas about potential KPI-improving changes, run new tests and start the process all over again.
This is how you create a cycle of continuous optimization – the core of a marketing or advertising strategy that treats the audience’s preferences as one of the top priorities.
Wrapping up: key A/B testing insights
Now that we’ve gone through the basics, let’s recap the steps required for effective A/B testing:
- Understand the situation: analyze historical and current performance data to find areas to improve and weak spots to address.
- Prioritize variables for testing: develop a hypothesis about which factors are more likely to improve the results measured in specific KPIs (like a conversion rate). As you need to test one variable at a time, choose it based on its importance level.
- Run your tests for the right sample size for a specific, sufficient, and limited period of time. Track the results as you do it, but don’t end your tests prematurely to minimize the influence of random factors.
- Analyze test results once you finish it, make conclusions based on the data you get, and adjust your marketing or advertising strategy accordingly.
- Test again when necessary: you’ll always have to modify your strategies based on changing circumstances and audience preferences, so test repeatedly and consistently.
Hopefully, now you understand what A/B testing is, why you need to do it for achieving better results with your advertising and marketing campaigns, and how to do it properly. Keep testing and see how your performance gets better - everything is possible if you follow the recommendations in our article.